14 Introduction to Infectious Diseases
Learning Objectives
- Learn about the dynamics of infectious diseases and their impact on human health
- Understand the role of mathematical models in predicting infectious disease outbreaks
- Explore the components of infectious disease models and their implications
“Several infectious diseases are emerging and threatening human health worldwide. The burden of infectious diseases is undeniably a global issue, causing millions of deaths annually.”1
The advent of machine learning (ML) has revolutionised the field of infectious disease research by providing robust tools for predicting outbreaks and understanding the dynamics of spread. In this chapter, we will enhance our understanding of these diseases and look at the effects on Disability-Adjusted Life Years (DALYs) by applying machine learning and data visualisation techniques learned in previous chapters (Chapter 6 and Chapter 10). To further improve the knowledge of the impact of infectious diseases on global health, we will explore how integrating ML models can improve the accuracy of disease burden estimations and provide valuable insights into the impact of infectious diseases on public health.
14.1 Infectious Diseases the Invisible Enemies
Emerging infectious diseases are a global concern, causing millions of deaths annually. Understanding their behaviour and predicting outbreaks is fundamental not only for public health but also for advancing prediction techniques that can be applied in other fields.
Microorganisms, including bacteria and viruses, adapt and evolve at a rate much faster than humans. For example, the generation time for bacteria can be as short as 20–30 minutes, while viruses can replicate in even shorter time frames. This rapid adaptation allows pathogens to evolve quickly, developing resistance to treatments and evading the host’s immune system.
Infectious diseases follow a multi-stage progression that begins when the infective agent, whether viral, bacterial, or parasitic, begins to thrive and multiply throughout the body2 and the process of infection starts. The rate at which the pathogen proliferates varies significantly depending on the type of organism involved. Each infectious disease has a unique incubation period, which is the interval between the initial establishment of the pathogen in the host and the onset of symptoms.
The incubation period can range from a few hours to several months, influenced by factors such as the pathogen’s growth rate, the host’s immune response, and the route of transmission. For example, the incubation period for the influenza virus is typically 1 to 4 days, whereas for diseases like hepatitis B, it can be as long as 6 months. Understanding the incubation period helps in identifying the time frame for potential exposure.
Several factors influence an individual’s susceptibility to infection, including:
- Infection dose (quantity of invading germs)
- Virulence (the ability of the organism to cause disease)
- Immune status (the condition of the body’s immune system)
- Transmission route (contact with the source of infection for contagious diseases)
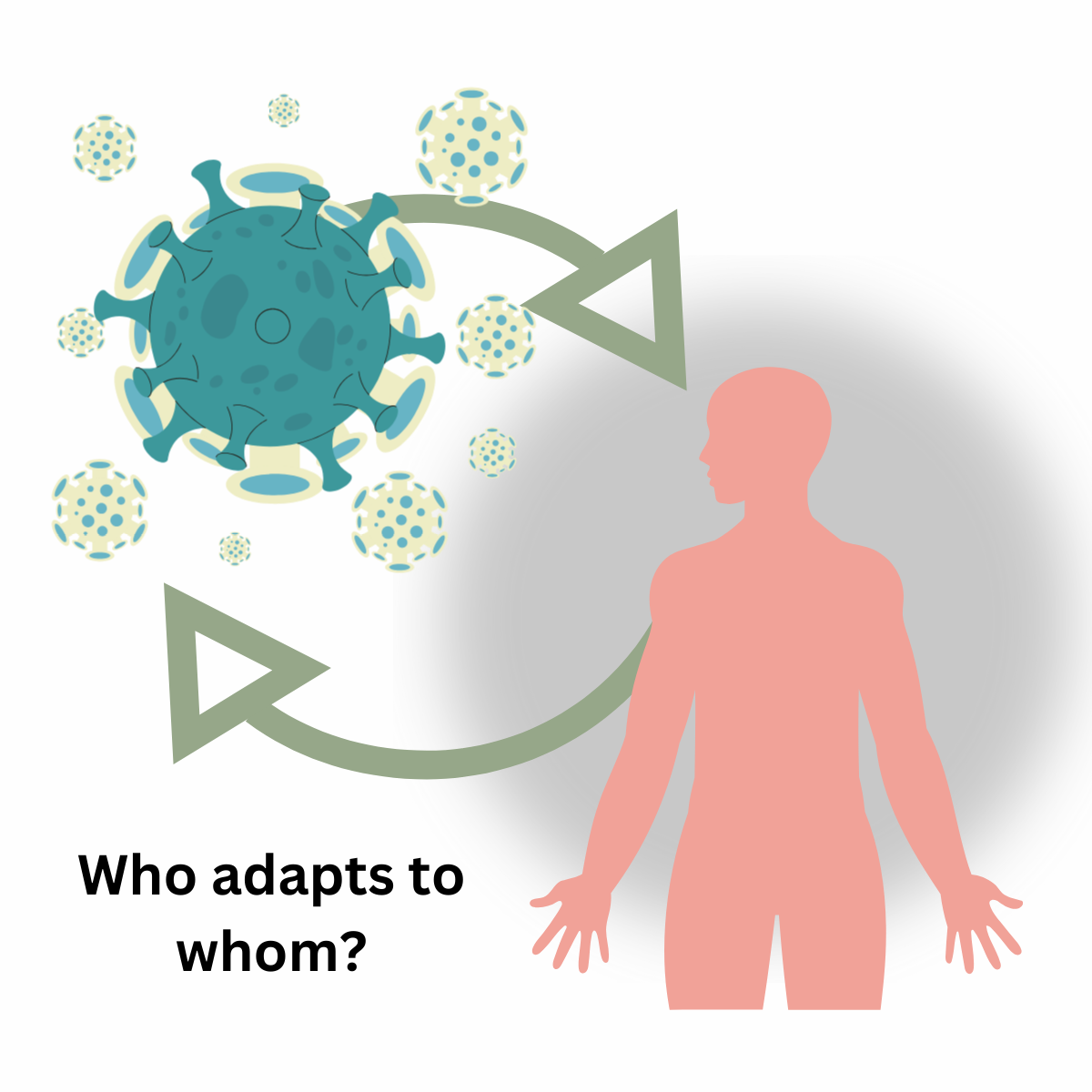
Viruses, word derived from the Latin word for “poisonous substance”, are intracellular parasites that can only replicate within living host cells. Their sizes range from 20 to 400 nm in diameter and can only be observed with an electron microscope. Outside of a living cell, a virus is a dormant particle of various shapes. Once inside a cell, it replicates, often killing the cell or altering its functions.
The following seven diseases are all caused by an infectious agent such as virus or bacteria, generally cause acute symptoms, ranging from mild to severe, and require prompt medical attention to prevent complications and further spread:
- Acute Respiratory Infection (ARI)
- Covid-19
- Dengue
- Influenza/Influenza-Like Illness (ILI)
- Malaria
- West Nile Virus
- Zika
These diseases are transmitted through various means and can be grouped by transmission methods:
- Vector-Borne: Dengue, Malaria, West Nile Virus, and Zika are primarily spread through mosquito bites.
- Respiratory Droplets: ARI, COVID-19, and Influenza/ILI are transmitted via respiratory droplets when infected individuals cough or sneeze.
14.2 Mathematical Models for Infectious Diseases
The application of mathematical models to infectious diseases dates back over a century, with significant contributions from pioneers such as Kermack and McKendrick, who established the foundations of the subject3. Their work introduced the concept of categorising individuals based on their epidemiological status: susceptible, infected, and recovered.
14.2.1 The SIR Model
One of the simplest and most fundamental epidemiological models, the SIR model, to which we had a quick look in the previous chapters (Chapter 6 and Chapter 7), is based on these three compartments and uses a system of differential equations to describe how individuals move between these compartments based on infection rate and the recovery rate. These parameters help predict the epidemic’s progression, showing how the number of susceptible individuals decreases as the number of infected individuals increases, eventually leading to recovery and a decline in new infections, as shown in Equation 6.1.
More complex models, includes:
- SEIR Model: This model introduces an exposed (E) compartment, which represents individuals who have been infected but are not yet infectious. This compartmentalization is particularly useful for diseases with significant incubation periods (e.g., COVID-19). Practical applications are in Section 6.3.2 and Section 15.3 .
- SIS Model: In this model, individuals who recover from infection do not gain lasting immunity, meaning they return to the susceptible class and can become reinfected.
- MSIR Model: In some diseases, such as measles, maternal antibodies provide temporary immunity to newborns. The M (maternal immunity) compartment is used in such cases.
14.3 Components of Infectious Disease Models
- Infection Rate: This parameter, often denoted as \beta (beta), controls how quickly the susceptible population becomes infected. It depends on factors such as contact rate and the probability of transmission per contact. It is the rate at which individuals move from the susceptible compartment to the infected compartment. The infection rate is proportional to the product of susceptible and infected individuals4.
\beta = \text{Contact Rate} \times \text{Probability of Transmission per Contact} \tag{14.1}
- Recovery Rate: Denoted by \gamma (gamma), this defines the rate at which infected individuals recover and either gain immunity or become susceptible again (depending on the model). The average duration of infection is the reciprocal of the recovery rate.
\gamma = \frac{1}{\text{Average Duration of Infection}} \tag{14.2}
- Incubation Period: In models like SEIR, the incubation period is the average time that exposed individuals take before they become infectious. This is a critical factor in diseases like COVID-19 and Ebola. The incubation period is the reciprocal of the rate at which individuals move from the exposed compartment to the infected compartment. \sigma is the rate at which individuals move from the exposed compartment to the infected compartment.
\text{Incubation Period} = \frac{1}{\sigma} \tag{14.3}
- Transmission Rate: The transmission rate often depends on how a disease spreads—whether it’s through respiratory droplets, direct contact, vectors like mosquitoes, or other means. Transmission rates are also influenced by human behaviours, such as hygiene practices and social distancing measures. The transmission rate is the product of the probability of transmission per contact and the number of contacts. \beta is the probability of transmission per contact, and the number of contacts is the average number of people an infected individual comes into contact with.
\text{Transmission Rate} = \beta \times \text{Number of Contacts} \tag{14.4}
- Reproduction Ratio (R_0): A key metric that indicates the average number of secondary cases generated by one infectious individual in a fully susceptible population is the basic reproduction ratio (R_0). This value is calculated using the formula in Equation 14.5. \beta is the transmission rate and \gamma is the recovery rate. As the ratio of the transmission rate to the recovery rate, R_0 provides a measure of the disease’s ability to spread.
R_0 = \frac{\beta}{\gamma} \tag{14.5}
In summary, the SIR model is a simple yet powerful tool for understanding the dynamics of infectious diseases. By tracking the movement of individuals between susceptible, infected, and recovered compartments, the model can predict the course of an epidemic and help public health officials make informed decisions about disease control measures. The value of R_0 determines the epidemic threshold:
\text{If } R_0 \left\{\begin{matrix} \begin{aligned} >1 = & \text{ Epidemic}\\ <1 = & \text{ End of Infection Transmission} \end{aligned} \end{matrix}\right. \tag{14.6}
To accounts for changes in the population’s immunity, the effective reproduction number (R_{eff}) is calculated on a susceptible population which is not completely susceptible, and value of R_{eff} results less than R0 due to the presence of immune individuals in the population.
Another critical concept in infectious disease is the herd immunity, which refers to the indirect protection from infectious diseases that occurs when a large percentage of a population becomes immune to the infection, either through vaccination or previous infections. The herd immunity is reached when the effective reproduction number is less than 1, and the disease stops spreading.
The SIR model shows the dynamics of an epidemic by looking at how it grows and eventually declines. Initially, the number of cases rises exponentially, leading to a peak, but as the susceptible population start reducing in number due to various factors, the growth rate slows with subsequent decline.
14.4 Advancements and Extensions
Mathematical modelling has evolved to include more complex factors such as age structure, stochasticity, and spatial dynamics. Age-structured models, for example, consider how different age groups interact and contribute to the spread of diseases, which is particularly important for diseases like measles or COVID-19. Stochastic models account for random events that can influence the course of an epidemic, such as the introduction of the disease into a new population.
The use of machine learning algorithms such as decision trees, random forests, support vector machines, and deep-learning networks such as Long short-term memory (LSTM) models, effectively improve the identification of patterns and trends that may not be obvious with mechanistic models. These models are able to improve prediction accuracy working smoothly with large datasets.
Combining models and data sources enhances prediction accuracy, various models and techniques can be applied to reduce bias and the risk of overfitting. For instance, ensemble learning combines the predictions of multiple models to improve accuracy. In this context, we will explore how machine learning can predict infectious disease outbreaks and their impacts on human health, ultimately aiming to reduce the burden of disease.
Another significant aspect to consider is the emerging use of transfer learning, which involves applying knowledge gained from one predictive task to another. This approach is especially useful when data is limited and models need to be adapted. Although relatively under-explored in infectious disease research, transfer learning holds significant promise for improving predictions in areas with scarce data. By leveraging information from related tasks, this technique can enhance model performance, leading to more accurate and reliable predictions in public health scenarios.5
14.5 The Impact on DALYs
To understand the magnitude of infectious diseases impacts on DALYs, we can simply consider the DALYs rate of change. The percentage change in total DALYs and DALYs due to infectious diseases, in general or for a specific infective virus such as COVID19, allows us to assess the impact on the overall burden of disease. In the case of COVID19 for example, the percentage change in DALYs due to COVID19 can explain how this virus affected global health and produced excess mortality and morbidity.
\text{Percent change in DALYs} = \frac{\text{DALYs due to Infectious Diseases}}{\text{Total DALYs}} \times 100 \tag{14.7}
Where the DALYs = \sum_{i=1}^{n}{(YLD_i + YLL_i)}, YLD and YLL are the years lived with disability and the years of life lost respectively.
This percentage change provides a measure of the impact of infectious diseases on the overall burden of disease. The DALYs due to infectious diseases can be calculated as the sum of the DALYs for each disease, and the total DALYs can be calculated as the sum of the DALYs for all diseases. The percentage change in DALYs due to infectious diseases can then be calculated as the ratio of the DALYs due to infectious diseases to the total DALYs, multiplied by 100.
Furthermore, the use of machine learning models used to predict the variation of number of DALYs due to infectious diseases over time can be a valuable tool to understand the impact of infectious diseases on global health. Two approaches can be valued:
DALYs as a function of the socio-demographic index (SDI): A composite index of the average income per person, educational attainment, and total fertility rate. The model function can be expressed as: DALY_{id}= f(SDI)+\epsilon \tag{14.8} where is the number of DALYs is the response variable, SDI the socio-demographic index acting as predictor, f(.) is the function that relates the number of DALYs to the socio-demographic index, and \epsilon is the error term.
DALYs as a function of the human development index (HDI): A composite index of life expectancy, education, and per capita income indicators. The model function can be expressed as: DALY_{id}= f(HDI)+\epsilon \tag{14.9} where is the number of DALYs is the response variable, HDI the human development index where is the number of DALYs is the response variable, HDI the socio-demographic index acting as predictor, f(.) is the function that relates the number of DALYs to the socio-demographic index, and \epsilon is the error term. Big data analytics with machine learning analysis are used to classify the patterns of global disease burden by human development index (HDI) to have a better understanding of DALYs caused by infectious diseases such as COVID19 given different levels of HDI.
This can help us to understand the trends and patterns of infectious diseases and their impact on global health.
Omar Enzo Santangelo et al., “Machine Learning and Prediction of Infectious Diseases: A Systematic Review,” Machine Learning and Knowledge Extraction 5, no. 1 (March 2023): 175–98, doi:10.3390/make5010013.↩︎
Lyle D. Broemeling, Bayesian Analysis of Infectious Diseases: COVID-19 and Beyond (New York: Chapman; Hall/CRC, 2021), doi:10.1201/9781003125983.↩︎
M. J. Keeling and L. Danon, “Mathematical Modelling of Infectious Diseases,” British Medical Bulletin 92, no. 1 (December 1, 2009): 33–42, doi:10.1093/bmb/ldp038.↩︎
Theodore Kolokolnikov and David Iron, “Law of Mass Action and Saturation in SIR Model with Application to Coronavirus Modelling,” Infectious Disease Modelling 6 (November 16, 2020): 91–97, doi:10.1016/j.idm.2020.11.002.↩︎
Kirstin Roster, Colm Connaughton, and Francisco A. Rodrigues, “Forecasting New Diseases in Low-Data Settings Using Transfer Learning,” Chaos, Solitons, and Fractals 161 (August 2022): 112306, doi:10.1016/j.chaos.2022.112306.↩︎